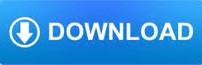
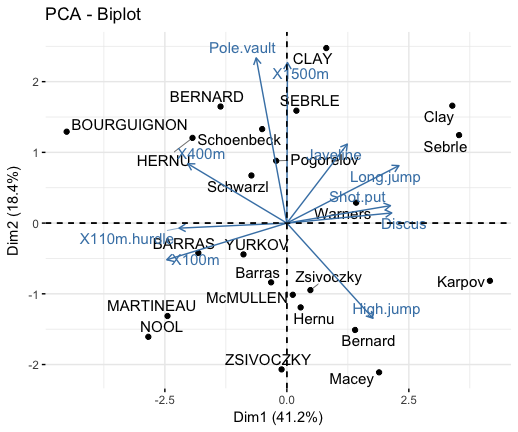
He gets a 1.5% commission on the selling price hence will grab you the best deal.
#Pca data iformat in r crack#
You are thoroughly confused and in good faith approached a real-estate agent to help you crack a good deal. Some say the housing market is booming and others believe it’s a bust. You get mixed messages from different sources about the state of the real-estate market. You want to sell your house that you had purchased 8 years ago. Information Asymmetry & Regression Models However, before we start building regression models let me highlight the importance of information in pricing and also explain how data science & regression creates a level playing field by eliminating information asymmetry. You can find all the parts of this case study at the following links: regression analysis case study example. In this part, you will learn nuances of regression modeling by building three different regression models and compare their results. We will also use results of the principal component analysis, discussed in the last part, to develop a regression model. This is a continuation of our case study example to estimate property pricing.
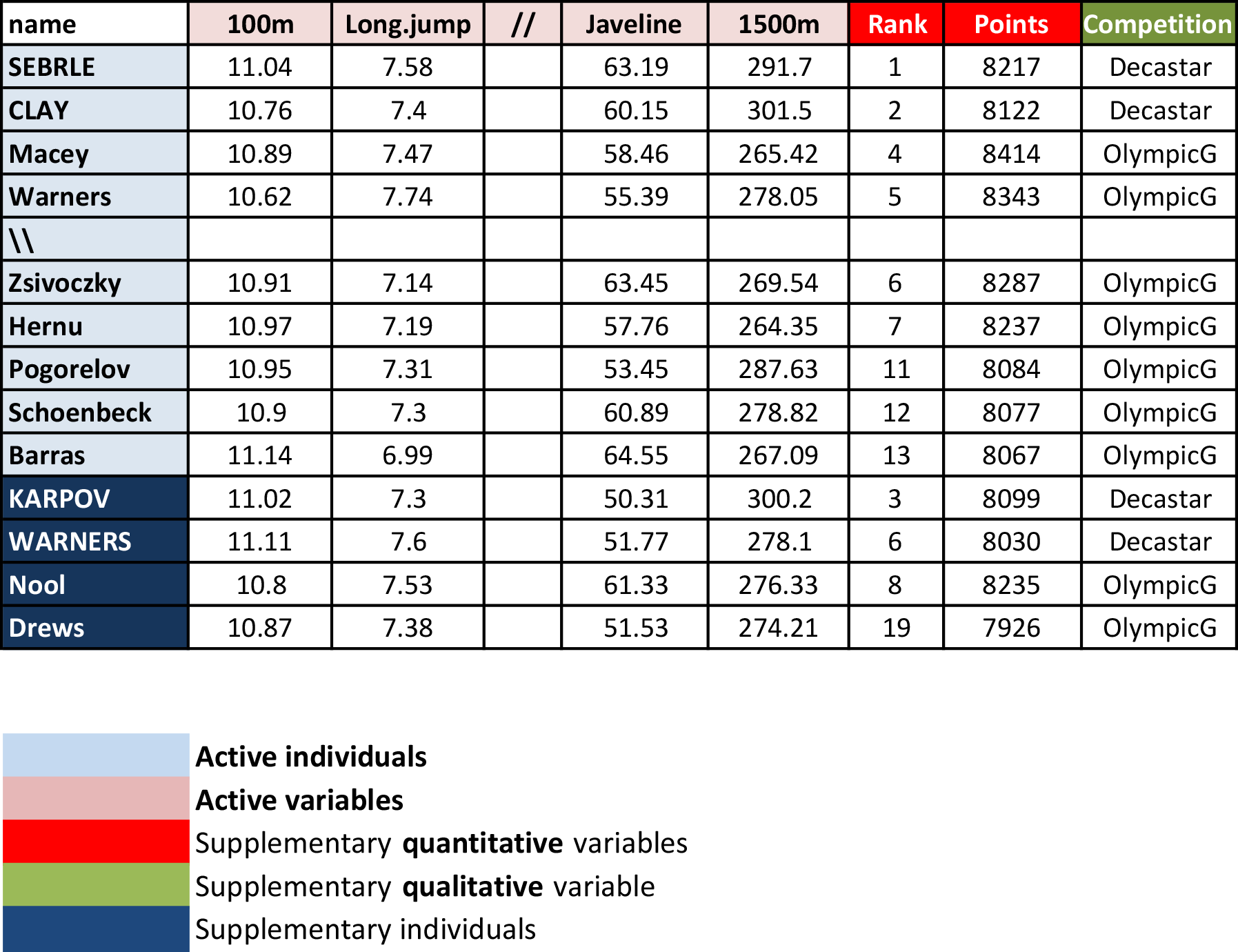
The toy data now looks like this:Ĭopy just the values (no sample or locus names) and paste into a text file, then remove the tabs between each column that Excel inserts.įinally, you may be asking why go through all of this trouble to use EIGENSOFT for PCA when you could also make one using the R package adegenet? I like EIGENSOFT because you can analyze the output with Tracy-Widom statistics (within the EIGENSOFT package) to identify which principal components are significant versus less rigorous ways such as observing the plateau of eigenvalues.Regression Models and Information Asymmetry – by Roopam (If you want to reference the formulas in the future, paste the values in a new tab.) Now copy the table (with values not formulas) again and use the transpose function. eigenstratgeno file, copy your Excel table, then paste the values to remove the formulas. Now we see that Locus1 of Sample2 has been coded as missing data (9) at all alleles.įinally, you need to make the three files (.eigenstratgeno. Since missing data should be missing in both columns in the original double column data (Tab 1), we only need to evaluate the first column, and assign it a value of 9 if it fulfills the if/then statement. Blank cells can be coded in Excel as empty quotes (e.g. To do this, we can still use the same idea as before by adding another if/then statement, but this time it will evaluate if the cell in the original data (Tab 1) is blank. However, we still have to account for the missing data. Yay! There are now counts for all of the alleles. Therefore, you can add (+) an additional if/then statement to account for the second allele in the second column, like this: You should have noticed that when you do this, you are only accounting for the first allele.
#Pca data iformat in r full#
Once formulas have been written for the first sample, you can drag the equations down to populate the full table. Write formulas for each locus_allele combination for the first sample, making sure to lock the right hand of the equation (allele value to compare the data to) using dollar signs before the row and column identifiers. Specifically, if the allele in the first column for the locus of interest was equal to the allele in the header row, then populate that cell with the value of 1 if the values in Tab 1 and Tab 2 are not equal, then populate the cell with a 0.

While this may be simple enough to do my hand for toy datasets, it is much easier (and less error prone) to use formulas in Excel to populate the new table. The next step is to populate the new table with the number of alleles (0, 1, or 2) that each sample has for each locus_allele combination. Also remember that it is important to keep the sample order the same as in the two column format. In Excel, I set up a new tab with the paired locus and allele information. In the toy example there are four alleles for Locus1, and three alleles for Locus2. You need to convert your data so that each allele of a microsatellite in your dataset has its own column. Note that Sample2 is missing data at Locus1. Let’s start with our data in double column format (e.g.- STRUCTURE format). This post provides an in-eloquent way of doing the format conversion using Excel tables.
#Pca data iformat in r how to#
However, the input format is not all that clear for how to convert your data. For anyone that works with microsatellites, you no doubt noticed the paragraph that says you can use microsatellite data as the PCA input. PCA is a great way to identify both population structure and admixture relationships. At this point, who hasn’t read Patterson et al 2006 about population structure and eigenvector analysis? It’s a great paper as it introduced the EIGENSOFT package for analyzing genomic data using principal components analysis (PCA).
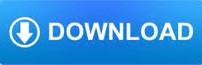